class: center, middle, inverse, title-slide # Multiple Regression II --- ## Last time * Introduction to multiple regression * Interpreting coefficient estimates * Estimating model fit * Significance tests (omnibus and coefficients) --- ## Today * Tolerance * Hierarchical regression/model comparison * Categorical predictors --- ```r library(here) stress.data = read.csv(here("data/stress.csv")) library(psych) describe(stress.data$Stress) ``` ``` ## vars n mean sd median trimmed mad min max range skew kurtosis se ## X1 1 118 5.18 1.88 5.27 5.17 1.65 0.62 10.32 9.71 0.08 0.22 0.17 ``` ```r mr.model <- lm(Stress ~ Support + Anxiety, data = stress.data) summary(mr.model) ``` ``` ## ## Call: ## lm(formula = Stress ~ Support + Anxiety, data = stress.data) ## ## Residuals: ## Min 1Q Median 3Q Max ## -4.1958 -0.8994 -0.1370 0.9990 3.6995 ## ## Coefficients: ## Estimate Std. Error t value Pr(>|t|) ## (Intercept) -0.31587 0.85596 -0.369 0.712792 ## Support 0.40618 0.05115 7.941 1.49e-12 *** ## Anxiety 0.25609 0.06740 3.799 0.000234 *** ## --- ## Signif. codes: 0 '***' 0.001 '**' 0.01 '*' 0.05 '.' 0.1 ' ' 1 ## ## Residual standard error: 1.519 on 115 degrees of freedom ## Multiple R-squared: 0.3556, Adjusted R-squared: 0.3444 ## F-statistic: 31.73 on 2 and 115 DF, p-value: 1.062e-11 ``` ??? Review here: * Omnibus test * Coefficient of determination * Adjust R squared * Resid standard error * Coefficient estimates --- ## Standard error of regression coefficient In the case of univariate regression: `$$\Large se_{b} = \frac{s_{Y}}{s_{X}}{\sqrt{\frac {1-r_{xy}^2}{n-2}}}$$` In the case of multiple regression: `$$\Large se_{b} = \frac{s_{Y}}{s_{X}}{\sqrt{\frac {1-R_{Y\hat{Y}}^2}{n-p-1}}} \sqrt{\frac {1}{1-R_{i.jkl...p}^2}}$$` - As N increases... - As variance explained increases... --- ## Tolerance `$$se_{b} = \frac{s_{Y}}{s_{X}}{\sqrt{\frac {1-R_{Y\hat{Y}}^2}{n-p-1}}} \sqrt{\frac {1}{1-R_{i.jkl...p}^2}}$$` - what cannot be explained in `\(X_i\)` by other predictors - Large tolerance (little overlap) means standard error will be small. - what does this mean for including a lot of variables in your model? --- ## Which variables to include - You goal should be to match the population model (theoretically) - Including many variables will not bias parameter estimates but will potentially increase degrees of freedom and standard errors; in other words, putting too many variables in your model may make it _more difficult_ to find a statistically significant result - But that's only the case if you add variables unrelated to Y or X; there are some cases in which adding the wrong variables can lead to spurious results. [Stay tuned for the lecture on causal models.] --- ## Methods for entering variables **Simultaneous**: Enter all of your IV's in a single model. `$$\large Y = b_0 + b_1X_1 + b_2X_2 + b_3X_3$$` - The benefits to using this method is that it reduces researcher degrees of freedom, is a more conservative test of any one coefficient, and often the most defensible action (unless you have specific theory guiding a hierarchical approach). --- ## Methods for entering variables **Hierarchically**: Build a sequence of models in which every successive model includes one more (or one fewer) IV than the previous. `$$\large Y = b_0 + e$$` `$$\large Y = b_0 + b_1X_1 + e$$` `$$\large Y = b_0 + b_1X_1 + b_2X_2 + e$$` `$$\large Y = b_0 + b_1X_1 + b_2X_2 + b_3X_3 + e$$` This is known as **hierarchical regression**. (Note that this is different from Hierarchical Linear Modelling or HLM [which is often called Multilevel Modeling or MLM].) Hierarchical regression is a subset of **model comparison** techniques. --- ## Hierarchical regression / Model Comparison **Model comparison:** Comparing how well two (or more) models fit the data in order to determine which model is better. If we're comparing nested models by incrementally adding or subtracting variables, this is known as hierarchical regression. - Multiple models are calculated - Each predictor (or set of predictors) is assessed in terms of what it adds (in terms of variance explained) at the time it is entered - Order is dependent on an _a priori_ hypothesis --- 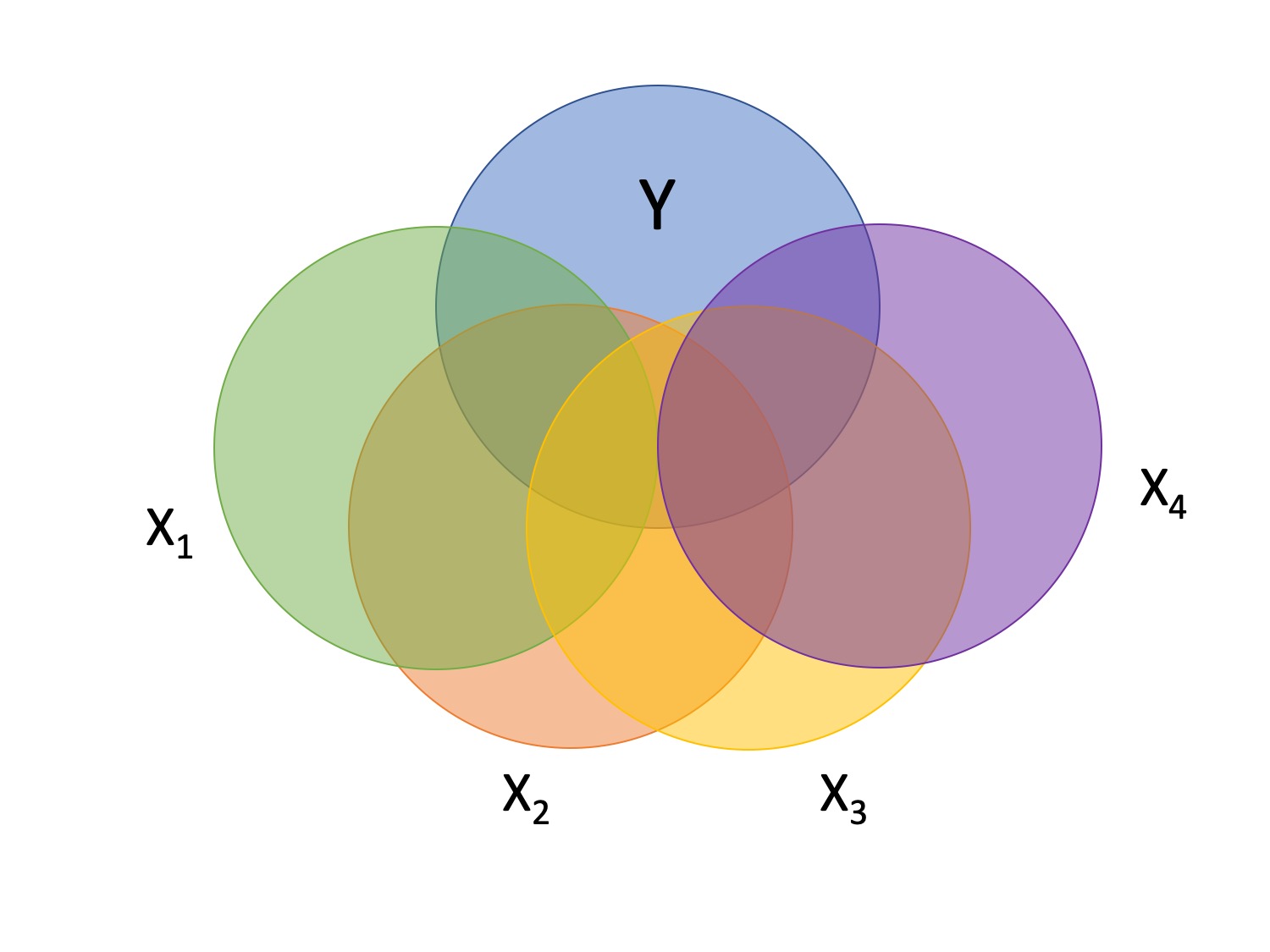 --- ## R-square change - distributed as an F `$$F(p.new, N - 1 - p.all) = \frac {R_{m.2}^2- R_{m.1}^2} {1-R_{m.2}^2} (\frac {N-1-p.all}{p.new})$$` - can also be written in terms of SSresiduals --- ## Model comparisons ```r m.1 <- lm(Stress ~ Support, data = stress.data) m.2 <- lm(Stress ~ Support + Anxiety, data = stress.data) anova(m.1, m.2) ``` ``` ## Analysis of Variance Table ## ## Model 1: Stress ~ Support ## Model 2: Stress ~ Support + Anxiety ## Res.Df RSS Df Sum of Sq F Pr(>F) ## 1 116 298.72 ## 2 115 265.41 1 33.314 14.435 0.0002336 *** ## --- ## Signif. codes: 0 '***' 0.001 '**' 0.01 '*' 0.05 '.' 0.1 ' ' 1 ``` --- ## model comparisons ```r anova(m.1) ``` ``` ## Analysis of Variance Table ## ## Response: Stress ## Df Sum Sq Mean Sq F value Pr(>F) ## Support 1 113.15 113.151 43.939 1.12e-09 *** ## Residuals 116 298.72 2.575 ## --- ## Signif. codes: 0 '***' 0.001 '**' 0.01 '*' 0.05 '.' 0.1 ' ' 1 ``` --- ## model comparisons ```r anova(m.2) ``` ``` ## Analysis of Variance Table ## ## Response: Stress ## Df Sum Sq Mean Sq F value Pr(>F) ## Support 1 113.151 113.151 49.028 1.807e-10 *** ## Anxiety 1 33.314 33.314 14.435 0.0002336 *** ## Residuals 115 265.407 2.308 ## --- ## Signif. codes: 0 '***' 0.001 '**' 0.01 '*' 0.05 '.' 0.1 ' ' 1 ``` --- ## model comparisons ``` ## ## Call: ## lm(formula = Stress ~ Support + Anxiety, data = stress.data) ## ## Residuals: ## Min 1Q Median 3Q Max ## -4.1958 -0.8994 -0.1370 0.9990 3.6995 ## ## Coefficients: ## Estimate Std. Error t value Pr(>|t|) ## (Intercept) -0.31587 0.85596 -0.369 0.712792 ## Support 0.40618 0.05115 7.941 1.49e-12 *** ## Anxiety 0.25609 0.06740 3.799 0.000234 *** ## --- ## Signif. codes: 0 '***' 0.001 '**' 0.01 '*' 0.05 '.' 0.1 ' ' 1 ## ## Residual standard error: 1.519 on 115 degrees of freedom ## Multiple R-squared: 0.3556, Adjusted R-squared: 0.3444 ## F-statistic: 31.73 on 2 and 115 DF, p-value: 1.062e-11 ``` --- ## model comparisons ``` ## ## Call: ## lm(formula = Stress ~ Support, data = stress.data) ## ## Residuals: ## Min 1Q Median 3Q Max ## -3.8215 -1.2145 -0.1796 1.0806 3.4326 ## ## Coefficients: ## Estimate Std. Error t value Pr(>|t|) ## (Intercept) 2.56046 0.42189 6.069 1.66e-08 *** ## Support 0.30006 0.04527 6.629 1.12e-09 *** ## --- ## Signif. codes: 0 '***' 0.001 '**' 0.01 '*' 0.05 '.' 0.1 ' ' 1 ## ## Residual standard error: 1.605 on 116 degrees of freedom ## Multiple R-squared: 0.2747, Adjusted R-squared: 0.2685 ## F-statistic: 43.94 on 1 and 116 DF, p-value: 1.12e-09 ``` --- ## model comparisons ```r m.0 <- lm(Stress ~ 1, data = stress.data) m.1 <- lm(Stress ~ Support, data = stress.data) anova(m.0, m.1) ``` ``` ## Analysis of Variance Table ## ## Model 1: Stress ~ 1 ## Model 2: Stress ~ Support ## Res.Df RSS Df Sum of Sq F Pr(>F) ## 1 117 411.87 ## 2 116 298.72 1 113.15 43.939 1.12e-09 *** ## --- ## Signif. codes: 0 '***' 0.001 '**' 0.01 '*' 0.05 '.' 0.1 ' ' 1 ``` --- ## partitioning the variance - It doesn't make sense to ask how much variance a variable explains (unless you qualify the association) `$$R_{Y.1234...p}^2 = r_{Y1}^2 + r_{Y(2.1)}^2 + r_{Y(3.21)}^2 + r_{Y(4.321)}^2 + ...$$` - In other words: order matters! --- ## Categorical predictors One of the benefits of using regression (instead of partial correlations) is that it can handle both continuous and categorical predictors and allows for using both in the same model. Categorical predictors with more than two levels are broken up into several smaller variables. In doing so, we take variables that don't have any inherent numerical value to them (i.e., nominal and ordinal variables) and ascribe meaningful numbers that allow for us to calculate meaningful statistics. You can choose just about any numbers to represent your categorical variable. However, there are several commonly used methods that result in very useful statistics. --- ## Dummy coding In dummy coding, one group is selected to be a reference group. From your single nominal variable with *K* levels, `\(K-1\)` dummy code variables are created; for each new dummy code variable, one of the non-reference groups is assigned 1; all other groups are assigned 0. .pull-left[ | Occupation | D1 | D2 | |:----------:|:--:|:--:| |Engineer | 0 | 0 | |Teacher | 1 | 0 | |Doctor | 0 | 1 | The dummy codes are entered as IV's in the regression equation. ] -- .pull-right[ |Person | Occupation | D1 | D2 | |:-----|:----------:|:--:|:--:| |Billy |Engineer | 0 | 0 | |Susan |Teacher | 1 | 0 | |Michael |Teacher | 1 | 0 | |Molly |Engineer | 0 | 0 | |Katie |Doctor | 0 | 1 | ] --- ### Example Solomon’s paradox describes the tendency for people to reason more wisely about other people’s problems compared to their own. One potential explanation for this paradox is that people tend to view other people’s problems from a more psychologically distant perspective, whereas they view their own problems from a psychologically immersed perspective. To test this possibility, researchers asked romantically-involved participants to think about a situation in which their partner cheated on them (self condition) or a friend’s partner cheated on their friend (other condition). Participants were also instructed to take a first-person perspective (immersed condition) by using pronouns such as I and me, or a third-person perspective (distanced condition) by using pronouns such as he and her. ```r library(here) solomon = read.csv(here("data/solomon.csv")) ``` .small[Grossmann, I., & Kross, E. (2014). Exploring Solomon’s paradox: Self-distancing eliminates self-other asymmetry in wise reasoning about close relationships in younger and older adults. _Psychological Science, 25_, 1571-1580.] --- ```r psych::describe(solomon[,c("ID", "CONDITION", "WISDOM")], fast = T) ``` ``` ## vars n mean sd min max range se ## ID 1 120 64.46 40.98 1.00 168.00 167.00 3.74 ## CONDITION 2 120 2.46 1.12 1.00 4.00 3.00 0.10 ## WISDOM 3 115 0.01 0.99 -2.52 1.79 4.31 0.09 ``` -- .pull-left[ ```r library(knitr) library(kableExtra) head(solomon) %>% select(ID, CONDITION, WISDOM) %>% kable() %>% kable_styling() ``` ] .pull-right[ <table class="table" style="margin-left: auto; margin-right: auto;"> <thead> <tr> <th style="text-align:right;"> ID </th> <th style="text-align:right;"> CONDITION </th> <th style="text-align:right;"> WISDOM </th> </tr> </thead> <tbody> <tr> <td style="text-align:right;"> 1 </td> <td style="text-align:right;"> 3 </td> <td style="text-align:right;"> -0.2758939 </td> </tr> <tr> <td style="text-align:right;"> 6 </td> <td style="text-align:right;"> 4 </td> <td style="text-align:right;"> 0.4294921 </td> </tr> <tr> <td style="text-align:right;"> 8 </td> <td style="text-align:right;"> 4 </td> <td style="text-align:right;"> -0.0278587 </td> </tr> <tr> <td style="text-align:right;"> 9 </td> <td style="text-align:right;"> 4 </td> <td style="text-align:right;"> 0.5327150 </td> </tr> <tr> <td style="text-align:right;"> 10 </td> <td style="text-align:right;"> 2 </td> <td style="text-align:right;"> 0.6229979 </td> </tr> <tr> <td style="text-align:right;"> 12 </td> <td style="text-align:right;"> 2 </td> <td style="text-align:right;"> -1.9957813 </td> </tr> </tbody> </table> ] --- ```r solomon = solomon %>% mutate(dummy_2 = ifelse(CONDITION == 2, 1, 0), dummy_3 = ifelse(CONDITION == 3, 1, 0), dummy_4 = ifelse(CONDITION == 4, 1, 0)) solomon %>% select(ID, CONDITION, WISDOM, matches("dummy")) %>% kable() %>% kable_styling() ``` <table class="table" style="margin-left: auto; margin-right: auto;"> <thead> <tr> <th style="text-align:right;"> ID </th> <th style="text-align:right;"> CONDITION </th> <th style="text-align:right;"> WISDOM </th> <th style="text-align:right;"> dummy_2 </th> <th style="text-align:right;"> dummy_3 </th> <th style="text-align:right;"> dummy_4 </th> </tr> </thead> <tbody> <tr> <td style="text-align:right;"> 1 </td> <td style="text-align:right;"> 3 </td> <td style="text-align:right;"> -0.2758939 </td> <td style="text-align:right;"> 0 </td> <td style="text-align:right;"> 1 </td> <td style="text-align:right;"> 0 </td> </tr> <tr> <td style="text-align:right;"> 6 </td> <td style="text-align:right;"> 4 </td> <td style="text-align:right;"> 0.4294921 </td> <td style="text-align:right;"> 0 </td> <td style="text-align:right;"> 0 </td> <td style="text-align:right;"> 1 </td> </tr> <tr> <td style="text-align:right;"> 8 </td> <td style="text-align:right;"> 4 </td> <td style="text-align:right;"> -0.0278587 </td> <td style="text-align:right;"> 0 </td> <td style="text-align:right;"> 0 </td> <td style="text-align:right;"> 1 </td> </tr> <tr> <td style="text-align:right;"> 9 </td> <td style="text-align:right;"> 4 </td> <td style="text-align:right;"> 0.5327150 </td> <td style="text-align:right;"> 0 </td> <td style="text-align:right;"> 0 </td> <td style="text-align:right;"> 1 </td> </tr> <tr> <td style="text-align:right;"> 10 </td> <td style="text-align:right;"> 2 </td> <td style="text-align:right;"> 0.6229979 </td> <td style="text-align:right;"> 1 </td> <td style="text-align:right;"> 0 </td> <td style="text-align:right;"> 0 </td> </tr> <tr> <td style="text-align:right;"> 12 </td> <td style="text-align:right;"> 2 </td> <td style="text-align:right;"> -1.9957813 </td> <td style="text-align:right;"> 1 </td> <td style="text-align:right;"> 0 </td> <td style="text-align:right;"> 0 </td> </tr> <tr> <td style="text-align:right;"> 14 </td> <td style="text-align:right;"> 3 </td> <td style="text-align:right;"> -1.1514699 </td> <td style="text-align:right;"> 0 </td> <td style="text-align:right;"> 1 </td> <td style="text-align:right;"> 0 </td> </tr> <tr> <td style="text-align:right;"> 18 </td> <td style="text-align:right;"> 2 </td> <td style="text-align:right;"> -0.6912011 </td> <td style="text-align:right;"> 1 </td> <td style="text-align:right;"> 0 </td> <td style="text-align:right;"> 0 </td> </tr> <tr> <td style="text-align:right;"> 21 </td> <td style="text-align:right;"> 2 </td> <td style="text-align:right;"> 0.0053117 </td> <td style="text-align:right;"> 1 </td> <td style="text-align:right;"> 0 </td> <td style="text-align:right;"> 0 </td> </tr> <tr> <td style="text-align:right;"> 25 </td> <td style="text-align:right;"> 4 </td> <td style="text-align:right;"> 0.2863499 </td> <td style="text-align:right;"> 0 </td> <td style="text-align:right;"> 0 </td> <td style="text-align:right;"> 1 </td> </tr> <tr> <td style="text-align:right;"> 26 </td> <td style="text-align:right;"> 4 </td> <td style="text-align:right;"> -1.8217968 </td> <td style="text-align:right;"> 0 </td> <td style="text-align:right;"> 0 </td> <td style="text-align:right;"> 1 </td> </tr> <tr> <td style="text-align:right;"> 30 </td> <td style="text-align:right;"> 1 </td> <td style="text-align:right;"> -1.2823302 </td> <td style="text-align:right;"> 0 </td> <td style="text-align:right;"> 0 </td> <td style="text-align:right;"> 0 </td> </tr> <tr> <td style="text-align:right;"> 32 </td> <td style="text-align:right;"> 1 </td> <td style="text-align:right;"> -2.3358379 </td> <td style="text-align:right;"> 0 </td> <td style="text-align:right;"> 0 </td> <td style="text-align:right;"> 0 </td> </tr> <tr> <td style="text-align:right;"> 35 </td> <td style="text-align:right;"> 4 </td> <td style="text-align:right;"> 0.2710307 </td> <td style="text-align:right;"> 0 </td> <td style="text-align:right;"> 0 </td> <td style="text-align:right;"> 1 </td> </tr> <tr> <td style="text-align:right;"> 50 </td> <td style="text-align:right;"> 1 </td> <td style="text-align:right;"> 0.7179373 </td> <td style="text-align:right;"> 0 </td> <td style="text-align:right;"> 0 </td> <td style="text-align:right;"> 0 </td> </tr> <tr> <td style="text-align:right;"> 53 </td> <td style="text-align:right;"> 1 </td> <td style="text-align:right;"> -2.0595072 </td> <td style="text-align:right;"> 0 </td> <td style="text-align:right;"> 0 </td> <td style="text-align:right;"> 0 </td> </tr> <tr> <td style="text-align:right;"> 57 </td> <td style="text-align:right;"> 4 </td> <td style="text-align:right;"> -0.2327698 </td> <td style="text-align:right;"> 0 </td> <td style="text-align:right;"> 0 </td> <td style="text-align:right;"> 1 </td> </tr> <tr> <td style="text-align:right;"> 58 </td> <td style="text-align:right;"> 4 </td> <td style="text-align:right;"> 0.0214245 </td> <td style="text-align:right;"> 0 </td> <td style="text-align:right;"> 0 </td> <td style="text-align:right;"> 1 </td> </tr> <tr> <td style="text-align:right;"> 60 </td> <td style="text-align:right;"> 3 </td> <td style="text-align:right;"> 0.1112851 </td> <td style="text-align:right;"> 0 </td> <td style="text-align:right;"> 1 </td> <td style="text-align:right;"> 0 </td> </tr> <tr> <td style="text-align:right;"> 62 </td> <td style="text-align:right;"> 1 </td> <td style="text-align:right;"> -1.7895030 </td> <td style="text-align:right;"> 0 </td> <td style="text-align:right;"> 0 </td> <td style="text-align:right;"> 0 </td> </tr> <tr> <td style="text-align:right;"> 65 </td> <td style="text-align:right;"> 2 </td> <td style="text-align:right;"> 0.9330889 </td> <td style="text-align:right;"> 1 </td> <td style="text-align:right;"> 0 </td> <td style="text-align:right;"> 0 </td> </tr> <tr> <td style="text-align:right;"> 68 </td> <td style="text-align:right;"> 1 </td> <td style="text-align:right;"> -0.3152235 </td> <td style="text-align:right;"> 0 </td> <td style="text-align:right;"> 0 </td> <td style="text-align:right;"> 0 </td> </tr> <tr> <td style="text-align:right;"> 71 </td> <td style="text-align:right;"> 4 </td> <td style="text-align:right;"> 0.7765844 </td> <td style="text-align:right;"> 0 </td> <td style="text-align:right;"> 0 </td> <td style="text-align:right;"> 1 </td> </tr> <tr> <td style="text-align:right;"> 76 </td> <td style="text-align:right;"> 4 </td> <td style="text-align:right;"> 1.1960573 </td> <td style="text-align:right;"> 0 </td> <td style="text-align:right;"> 0 </td> <td style="text-align:right;"> 1 </td> </tr> <tr> <td style="text-align:right;"> 84 </td> <td style="text-align:right;"> 2 </td> <td style="text-align:right;"> 0.0248331 </td> <td style="text-align:right;"> 1 </td> <td style="text-align:right;"> 0 </td> <td style="text-align:right;"> 0 </td> </tr> <tr> <td style="text-align:right;"> 86 </td> <td style="text-align:right;"> 3 </td> <td style="text-align:right;"> 1.2175357 </td> <td style="text-align:right;"> 0 </td> <td style="text-align:right;"> 1 </td> <td style="text-align:right;"> 0 </td> </tr> <tr> <td style="text-align:right;"> 88 </td> <td style="text-align:right;"> 3 </td> <td style="text-align:right;"> 0.5025819 </td> <td style="text-align:right;"> 0 </td> <td style="text-align:right;"> 1 </td> <td style="text-align:right;"> 0 </td> </tr> <tr> <td style="text-align:right;"> 89 </td> <td style="text-align:right;"> 1 </td> <td style="text-align:right;"> -0.4693998 </td> <td style="text-align:right;"> 0 </td> <td style="text-align:right;"> 0 </td> <td style="text-align:right;"> 0 </td> </tr> <tr> <td style="text-align:right;"> 95 </td> <td style="text-align:right;"> 4 </td> <td style="text-align:right;"> 0.4821839 </td> <td style="text-align:right;"> 0 </td> <td style="text-align:right;"> 0 </td> <td style="text-align:right;"> 1 </td> </tr> <tr> <td style="text-align:right;"> 99 </td> <td style="text-align:right;"> 1 </td> <td style="text-align:right;"> -0.0352657 </td> <td style="text-align:right;"> 0 </td> <td style="text-align:right;"> 0 </td> <td style="text-align:right;"> 0 </td> </tr> <tr> <td style="text-align:right;"> 102 </td> <td style="text-align:right;"> 1 </td> <td style="text-align:right;"> 1.1155606 </td> <td style="text-align:right;"> 0 </td> <td style="text-align:right;"> 0 </td> <td style="text-align:right;"> 0 </td> </tr> <tr> <td style="text-align:right;"> 105 </td> <td style="text-align:right;"> 2 </td> <td style="text-align:right;"> 1.4556172 </td> <td style="text-align:right;"> 1 </td> <td style="text-align:right;"> 0 </td> <td style="text-align:right;"> 0 </td> </tr> <tr> <td style="text-align:right;"> 117 </td> <td style="text-align:right;"> 1 </td> <td style="text-align:right;"> NA </td> <td style="text-align:right;"> 0 </td> <td style="text-align:right;"> 0 </td> <td style="text-align:right;"> 0 </td> </tr> <tr> <td style="text-align:right;"> 122 </td> <td style="text-align:right;"> 2 </td> <td style="text-align:right;"> 0.4161299 </td> <td style="text-align:right;"> 1 </td> <td style="text-align:right;"> 0 </td> <td style="text-align:right;"> 0 </td> </tr> <tr> <td style="text-align:right;"> 143 </td> <td style="text-align:right;"> 1 </td> <td style="text-align:right;"> -1.3339417 </td> <td style="text-align:right;"> 0 </td> <td style="text-align:right;"> 0 </td> <td style="text-align:right;"> 0 </td> </tr> <tr> <td style="text-align:right;"> 145 </td> <td style="text-align:right;"> 4 </td> <td style="text-align:right;"> NA </td> <td style="text-align:right;"> 0 </td> <td style="text-align:right;"> 0 </td> <td style="text-align:right;"> 1 </td> </tr> <tr> <td style="text-align:right;"> 152 </td> <td style="text-align:right;"> 4 </td> <td style="text-align:right;"> 0.6508028 </td> <td style="text-align:right;"> 0 </td> <td style="text-align:right;"> 0 </td> <td style="text-align:right;"> 1 </td> </tr> <tr> <td style="text-align:right;"> 153 </td> <td style="text-align:right;"> 2 </td> <td style="text-align:right;"> -1.8543092 </td> <td style="text-align:right;"> 1 </td> <td style="text-align:right;"> 0 </td> <td style="text-align:right;"> 0 </td> </tr> <tr> <td style="text-align:right;"> 159 </td> <td style="text-align:right;"> 2 </td> <td style="text-align:right;"> -0.8511141 </td> <td style="text-align:right;"> 1 </td> <td style="text-align:right;"> 0 </td> <td style="text-align:right;"> 0 </td> </tr> <tr> <td style="text-align:right;"> 168 </td> <td style="text-align:right;"> 2 </td> <td style="text-align:right;"> 0.0029835 </td> <td style="text-align:right;"> 1 </td> <td style="text-align:right;"> 0 </td> <td style="text-align:right;"> 0 </td> </tr> <tr> <td style="text-align:right;"> 2 </td> <td style="text-align:right;"> 4 </td> <td style="text-align:right;"> 0.1340113 </td> <td style="text-align:right;"> 0 </td> <td style="text-align:right;"> 0 </td> <td style="text-align:right;"> 1 </td> </tr> <tr> <td style="text-align:right;"> 3 </td> <td style="text-align:right;"> 4 </td> <td style="text-align:right;"> -0.8836265 </td> <td style="text-align:right;"> 0 </td> <td style="text-align:right;"> 0 </td> <td style="text-align:right;"> 1 </td> </tr> <tr> <td style="text-align:right;"> 4 </td> <td style="text-align:right;"> 4 </td> <td style="text-align:right;"> 0.9063644 </td> <td style="text-align:right;"> 0 </td> <td style="text-align:right;"> 0 </td> <td style="text-align:right;"> 1 </td> </tr> <tr> <td style="text-align:right;"> 5 </td> <td style="text-align:right;"> 1 </td> <td style="text-align:right;"> 1.7905951 </td> <td style="text-align:right;"> 0 </td> <td style="text-align:right;"> 0 </td> <td style="text-align:right;"> 0 </td> </tr> <tr> <td style="text-align:right;"> 7 </td> <td style="text-align:right;"> 1 </td> <td style="text-align:right;"> -0.9868494 </td> <td style="text-align:right;"> 0 </td> <td style="text-align:right;"> 0 </td> <td style="text-align:right;"> 0 </td> </tr> <tr> <td style="text-align:right;"> 11 </td> <td style="text-align:right;"> 3 </td> <td style="text-align:right;"> 1.0372247 </td> <td style="text-align:right;"> 0 </td> <td style="text-align:right;"> 1 </td> <td style="text-align:right;"> 0 </td> </tr> <tr> <td style="text-align:right;"> 13 </td> <td style="text-align:right;"> 3 </td> <td style="text-align:right;"> -2.4860158 </td> <td style="text-align:right;"> 0 </td> <td style="text-align:right;"> 1 </td> <td style="text-align:right;"> 0 </td> </tr> <tr> <td style="text-align:right;"> 15 </td> <td style="text-align:right;"> 2 </td> <td style="text-align:right;"> 1.1166410 </td> <td style="text-align:right;"> 1 </td> <td style="text-align:right;"> 0 </td> <td style="text-align:right;"> 0 </td> </tr> <tr> <td style="text-align:right;"> 16 </td> <td style="text-align:right;"> 3 </td> <td style="text-align:right;"> 0.0412327 </td> <td style="text-align:right;"> 0 </td> <td style="text-align:right;"> 1 </td> <td style="text-align:right;"> 0 </td> </tr> <tr> <td style="text-align:right;"> 17 </td> <td style="text-align:right;"> 3 </td> <td style="text-align:right;"> 0.1183208 </td> <td style="text-align:right;"> 0 </td> <td style="text-align:right;"> 1 </td> <td style="text-align:right;"> 0 </td> </tr> <tr> <td style="text-align:right;"> 19 </td> <td style="text-align:right;"> 2 </td> <td style="text-align:right;"> -1.2353752 </td> <td style="text-align:right;"> 1 </td> <td style="text-align:right;"> 0 </td> <td style="text-align:right;"> 0 </td> </tr> <tr> <td style="text-align:right;"> 20 </td> <td style="text-align:right;"> 3 </td> <td style="text-align:right;"> 0.5182724 </td> <td style="text-align:right;"> 0 </td> <td style="text-align:right;"> 1 </td> <td style="text-align:right;"> 0 </td> </tr> <tr> <td style="text-align:right;"> 22 </td> <td style="text-align:right;"> 3 </td> <td style="text-align:right;"> 0.6202474 </td> <td style="text-align:right;"> 0 </td> <td style="text-align:right;"> 1 </td> <td style="text-align:right;"> 0 </td> </tr> <tr> <td style="text-align:right;"> 23 </td> <td style="text-align:right;"> 3 </td> <td style="text-align:right;"> -0.6130326 </td> <td style="text-align:right;"> 0 </td> <td style="text-align:right;"> 1 </td> <td style="text-align:right;"> 0 </td> </tr> <tr> <td style="text-align:right;"> 24 </td> <td style="text-align:right;"> 2 </td> <td style="text-align:right;"> 0.0114708 </td> <td style="text-align:right;"> 1 </td> <td style="text-align:right;"> 0 </td> <td style="text-align:right;"> 0 </td> </tr> <tr> <td style="text-align:right;"> 27 </td> <td style="text-align:right;"> 4 </td> <td style="text-align:right;"> 0.5735473 </td> <td style="text-align:right;"> 0 </td> <td style="text-align:right;"> 0 </td> <td style="text-align:right;"> 1 </td> </tr> <tr> <td style="text-align:right;"> 29 </td> <td style="text-align:right;"> 1 </td> <td style="text-align:right;"> -0.9486002 </td> <td style="text-align:right;"> 0 </td> <td style="text-align:right;"> 0 </td> <td style="text-align:right;"> 0 </td> </tr> <tr> <td style="text-align:right;"> 31 </td> <td style="text-align:right;"> 1 </td> <td style="text-align:right;"> 0.1183208 </td> <td style="text-align:right;"> 0 </td> <td style="text-align:right;"> 0 </td> <td style="text-align:right;"> 0 </td> </tr> <tr> <td style="text-align:right;"> 33 </td> <td style="text-align:right;"> 3 </td> <td style="text-align:right;"> -0.0208230 </td> <td style="text-align:right;"> 0 </td> <td style="text-align:right;"> 1 </td> <td style="text-align:right;"> 0 </td> </tr> <tr> <td style="text-align:right;"> 34 </td> <td style="text-align:right;"> 3 </td> <td style="text-align:right;"> 0.9004090 </td> <td style="text-align:right;"> 0 </td> <td style="text-align:right;"> 1 </td> <td style="text-align:right;"> 0 </td> </tr> <tr> <td style="text-align:right;"> 36 </td> <td style="text-align:right;"> 4 </td> <td style="text-align:right;"> 0.8704434 </td> <td style="text-align:right;"> 0 </td> <td style="text-align:right;"> 0 </td> <td style="text-align:right;"> 1 </td> </tr> <tr> <td style="text-align:right;"> 37 </td> <td style="text-align:right;"> 3 </td> <td style="text-align:right;"> 0.9556476 </td> <td style="text-align:right;"> 0 </td> <td style="text-align:right;"> 1 </td> <td style="text-align:right;"> 0 </td> </tr> <tr> <td style="text-align:right;"> 38 </td> <td style="text-align:right;"> 2 </td> <td style="text-align:right;"> 1.0240299 </td> <td style="text-align:right;"> 1 </td> <td style="text-align:right;"> 0 </td> <td style="text-align:right;"> 0 </td> </tr> <tr> <td style="text-align:right;"> 39 </td> <td style="text-align:right;"> 3 </td> <td style="text-align:right;"> -0.1556817 </td> <td style="text-align:right;"> 0 </td> <td style="text-align:right;"> 1 </td> <td style="text-align:right;"> 0 </td> </tr> <tr> <td style="text-align:right;"> 40 </td> <td style="text-align:right;"> 3 </td> <td style="text-align:right;"> 0.6229979 </td> <td style="text-align:right;"> 0 </td> <td style="text-align:right;"> 1 </td> <td style="text-align:right;"> 0 </td> </tr> <tr> <td style="text-align:right;"> 41 </td> <td style="text-align:right;"> 2 </td> <td style="text-align:right;"> -0.8691839 </td> <td style="text-align:right;"> 1 </td> <td style="text-align:right;"> 0 </td> <td style="text-align:right;"> 0 </td> </tr> <tr> <td style="text-align:right;"> 42 </td> <td style="text-align:right;"> 4 </td> <td style="text-align:right;"> 1.2319783 </td> <td style="text-align:right;"> 0 </td> <td style="text-align:right;"> 0 </td> <td style="text-align:right;"> 1 </td> </tr> <tr> <td style="text-align:right;"> 43 </td> <td style="text-align:right;"> 1 </td> <td style="text-align:right;"> -1.4556055 </td> <td style="text-align:right;"> 0 </td> <td style="text-align:right;"> 0 </td> <td style="text-align:right;"> 0 </td> </tr> <tr> <td style="text-align:right;"> 44 </td> <td style="text-align:right;"> 4 </td> <td style="text-align:right;"> 0.9341692 </td> <td style="text-align:right;"> 0 </td> <td style="text-align:right;"> 0 </td> <td style="text-align:right;"> 1 </td> </tr> <tr> <td style="text-align:right;"> 45 </td> <td style="text-align:right;"> 4 </td> <td style="text-align:right;"> -0.2287715 </td> <td style="text-align:right;"> 0 </td> <td style="text-align:right;"> 0 </td> <td style="text-align:right;"> 1 </td> </tr> <tr> <td style="text-align:right;"> 46 </td> <td style="text-align:right;"> 1 </td> <td style="text-align:right;"> -0.2903366 </td> <td style="text-align:right;"> 0 </td> <td style="text-align:right;"> 0 </td> <td style="text-align:right;"> 0 </td> </tr> <tr> <td style="text-align:right;"> 47 </td> <td style="text-align:right;"> 2 </td> <td style="text-align:right;"> 0.7034946 </td> <td style="text-align:right;"> 1 </td> <td style="text-align:right;"> 0 </td> <td style="text-align:right;"> 0 </td> </tr> <tr> <td style="text-align:right;"> 48 </td> <td style="text-align:right;"> 3 </td> <td style="text-align:right;"> 0.7551061 </td> <td style="text-align:right;"> 0 </td> <td style="text-align:right;"> 1 </td> <td style="text-align:right;"> 0 </td> </tr> <tr> <td style="text-align:right;"> 49 </td> <td style="text-align:right;"> 3 </td> <td style="text-align:right;"> -0.5291273 </td> <td style="text-align:right;"> 0 </td> <td style="text-align:right;"> 1 </td> <td style="text-align:right;"> 0 </td> </tr> <tr> <td style="text-align:right;"> 51 </td> <td style="text-align:right;"> 1 </td> <td style="text-align:right;"> 0.7262208 </td> <td style="text-align:right;"> 0 </td> <td style="text-align:right;"> 0 </td> <td style="text-align:right;"> 0 </td> </tr> <tr> <td style="text-align:right;"> 52 </td> <td style="text-align:right;"> 2 </td> <td style="text-align:right;"> 0.6108835 </td> <td style="text-align:right;"> 1 </td> <td style="text-align:right;"> 0 </td> <td style="text-align:right;"> 0 </td> </tr> <tr> <td style="text-align:right;"> 54 </td> <td style="text-align:right;"> 3 </td> <td style="text-align:right;"> -0.1134342 </td> <td style="text-align:right;"> 0 </td> <td style="text-align:right;"> 1 </td> <td style="text-align:right;"> 0 </td> </tr> <tr> <td style="text-align:right;"> 55 </td> <td style="text-align:right;"> 3 </td> <td style="text-align:right;"> 0.4150495 </td> <td style="text-align:right;"> 0 </td> <td style="text-align:right;"> 1 </td> <td style="text-align:right;"> 0 </td> </tr> <tr> <td style="text-align:right;"> 56 </td> <td style="text-align:right;"> 3 </td> <td style="text-align:right;"> 1.2991128 </td> <td style="text-align:right;"> 0 </td> <td style="text-align:right;"> 1 </td> <td style="text-align:right;"> 0 </td> </tr> <tr> <td style="text-align:right;"> 59 </td> <td style="text-align:right;"> 1 </td> <td style="text-align:right;"> -2.3324293 </td> <td style="text-align:right;"> 0 </td> <td style="text-align:right;"> 0 </td> <td style="text-align:right;"> 0 </td> </tr> <tr> <td style="text-align:right;"> 61 </td> <td style="text-align:right;"> 3 </td> <td style="text-align:right;"> -1.1745673 </td> <td style="text-align:right;"> 0 </td> <td style="text-align:right;"> 1 </td> <td style="text-align:right;"> 0 </td> </tr> <tr> <td style="text-align:right;"> 63 </td> <td style="text-align:right;"> 3 </td> <td style="text-align:right;"> 0.8560007 </td> <td style="text-align:right;"> 0 </td> <td style="text-align:right;"> 1 </td> <td style="text-align:right;"> 0 </td> </tr> <tr> <td style="text-align:right;"> 64 </td> <td style="text-align:right;"> 2 </td> <td style="text-align:right;"> -0.0486279 </td> <td style="text-align:right;"> 1 </td> <td style="text-align:right;"> 0 </td> <td style="text-align:right;"> 0 </td> </tr> <tr> <td style="text-align:right;"> 66 </td> <td style="text-align:right;"> 2 </td> <td style="text-align:right;"> 0.9532683 </td> <td style="text-align:right;"> 1 </td> <td style="text-align:right;"> 0 </td> <td style="text-align:right;"> 0 </td> </tr> <tr> <td style="text-align:right;"> 67 </td> <td style="text-align:right;"> 4 </td> <td style="text-align:right;"> NA </td> <td style="text-align:right;"> 0 </td> <td style="text-align:right;"> 0 </td> <td style="text-align:right;"> 1 </td> </tr> <tr> <td style="text-align:right;"> 69 </td> <td style="text-align:right;"> 2 </td> <td style="text-align:right;"> 0.8188319 </td> <td style="text-align:right;"> 1 </td> <td style="text-align:right;"> 0 </td> <td style="text-align:right;"> 0 </td> </tr> <tr> <td style="text-align:right;"> 70 </td> <td style="text-align:right;"> 4 </td> <td style="text-align:right;"> 1.6041250 </td> <td style="text-align:right;"> 0 </td> <td style="text-align:right;"> 0 </td> <td style="text-align:right;"> 1 </td> </tr> <tr> <td style="text-align:right;"> 72 </td> <td style="text-align:right;"> 2 </td> <td style="text-align:right;"> 0.9870285 </td> <td style="text-align:right;"> 1 </td> <td style="text-align:right;"> 0 </td> <td style="text-align:right;"> 0 </td> </tr> <tr> <td style="text-align:right;"> 73 </td> <td style="text-align:right;"> 4 </td> <td style="text-align:right;"> 0.1554896 </td> <td style="text-align:right;"> 0 </td> <td style="text-align:right;"> 0 </td> <td style="text-align:right;"> 1 </td> </tr> <tr> <td style="text-align:right;"> 74 </td> <td style="text-align:right;"> 1 </td> <td style="text-align:right;"> 0.3141548 </td> <td style="text-align:right;"> 0 </td> <td style="text-align:right;"> 0 </td> <td style="text-align:right;"> 0 </td> </tr> <tr> <td style="text-align:right;"> 75 </td> <td style="text-align:right;"> 1 </td> <td style="text-align:right;"> NA </td> <td style="text-align:right;"> 0 </td> <td style="text-align:right;"> 0 </td> <td style="text-align:right;"> 0 </td> </tr> <tr> <td style="text-align:right;"> 77 </td> <td style="text-align:right;"> 1 </td> <td style="text-align:right;"> -2.3046244 </td> <td style="text-align:right;"> 0 </td> <td style="text-align:right;"> 0 </td> <td style="text-align:right;"> 0 </td> </tr> <tr> <td style="text-align:right;"> 78 </td> <td style="text-align:right;"> 1 </td> <td style="text-align:right;"> 0.2277028 </td> <td style="text-align:right;"> 0 </td> <td style="text-align:right;"> 0 </td> <td style="text-align:right;"> 0 </td> </tr> <tr> <td style="text-align:right;"> 79 </td> <td style="text-align:right;"> 4 </td> <td style="text-align:right;"> 0.0545949 </td> <td style="text-align:right;"> 0 </td> <td style="text-align:right;"> 0 </td> <td style="text-align:right;"> 1 </td> </tr> <tr> <td style="text-align:right;"> 80 </td> <td style="text-align:right;"> 3 </td> <td style="text-align:right;"> -0.1217177 </td> <td style="text-align:right;"> 0 </td> <td style="text-align:right;"> 1 </td> <td style="text-align:right;"> 0 </td> </tr> <tr> <td style="text-align:right;"> 81 </td> <td style="text-align:right;"> 1 </td> <td style="text-align:right;"> -0.8641051 </td> <td style="text-align:right;"> 0 </td> <td style="text-align:right;"> 0 </td> <td style="text-align:right;"> 0 </td> </tr> <tr> <td style="text-align:right;"> 82 </td> <td style="text-align:right;"> 3 </td> <td style="text-align:right;"> 0.3524040 </td> <td style="text-align:right;"> 0 </td> <td style="text-align:right;"> 1 </td> <td style="text-align:right;"> 0 </td> </tr> <tr> <td style="text-align:right;"> 83 </td> <td style="text-align:right;"> 3 </td> <td style="text-align:right;"> 0.1565700 </td> <td style="text-align:right;"> 0 </td> <td style="text-align:right;"> 1 </td> <td style="text-align:right;"> 0 </td> </tr> <tr> <td style="text-align:right;"> 85 </td> <td style="text-align:right;"> 3 </td> <td style="text-align:right;"> 0.3430401 </td> <td style="text-align:right;"> 0 </td> <td style="text-align:right;"> 1 </td> <td style="text-align:right;"> 0 </td> </tr> <tr> <td style="text-align:right;"> 87 </td> <td style="text-align:right;"> 2 </td> <td style="text-align:right;"> 1.1792865 </td> <td style="text-align:right;"> 1 </td> <td style="text-align:right;"> 0 </td> <td style="text-align:right;"> 0 </td> </tr> <tr> <td style="text-align:right;"> 90 </td> <td style="text-align:right;"> 1 </td> <td style="text-align:right;"> 0.4329007 </td> <td style="text-align:right;"> 0 </td> <td style="text-align:right;"> 0 </td> <td style="text-align:right;"> 0 </td> </tr> <tr> <td style="text-align:right;"> 91 </td> <td style="text-align:right;"> 2 </td> <td style="text-align:right;"> -0.8083760 </td> <td style="text-align:right;"> 1 </td> <td style="text-align:right;"> 0 </td> <td style="text-align:right;"> 0 </td> </tr> <tr> <td style="text-align:right;"> 92 </td> <td style="text-align:right;"> 1 </td> <td style="text-align:right;"> 1.1427757 </td> <td style="text-align:right;"> 0 </td> <td style="text-align:right;"> 0 </td> <td style="text-align:right;"> 0 </td> </tr> <tr> <td style="text-align:right;"> 93 </td> <td style="text-align:right;"> 1 </td> <td style="text-align:right;"> 0.4101745 </td> <td style="text-align:right;"> 0 </td> <td style="text-align:right;"> 0 </td> <td style="text-align:right;"> 0 </td> </tr> <tr> <td style="text-align:right;"> 94 </td> <td style="text-align:right;"> 3 </td> <td style="text-align:right;"> 0.2387368 </td> <td style="text-align:right;"> 0 </td> <td style="text-align:right;"> 1 </td> <td style="text-align:right;"> 0 </td> </tr> <tr> <td style="text-align:right;"> 96 </td> <td style="text-align:right;"> 1 </td> <td style="text-align:right;"> -1.3751088 </td> <td style="text-align:right;"> 0 </td> <td style="text-align:right;"> 0 </td> <td style="text-align:right;"> 0 </td> </tr> <tr> <td style="text-align:right;"> 97 </td> <td style="text-align:right;"> 2 </td> <td style="text-align:right;"> 0.0834802 </td> <td style="text-align:right;"> 1 </td> <td style="text-align:right;"> 0 </td> <td style="text-align:right;"> 0 </td> </tr> <tr> <td style="text-align:right;"> 98 </td> <td style="text-align:right;"> 1 </td> <td style="text-align:right;"> -0.9282022 </td> <td style="text-align:right;"> 0 </td> <td style="text-align:right;"> 0 </td> <td style="text-align:right;"> 0 </td> </tr> <tr> <td style="text-align:right;"> 100 </td> <td style="text-align:right;"> 4 </td> <td style="text-align:right;"> 1.6584869 </td> <td style="text-align:right;"> 0 </td> <td style="text-align:right;"> 0 </td> <td style="text-align:right;"> 1 </td> </tr> <tr> <td style="text-align:right;"> 101 </td> <td style="text-align:right;"> 1 </td> <td style="text-align:right;"> -0.5150559 </td> <td style="text-align:right;"> 0 </td> <td style="text-align:right;"> 0 </td> <td style="text-align:right;"> 0 </td> </tr> <tr> <td style="text-align:right;"> 103 </td> <td style="text-align:right;"> 3 </td> <td style="text-align:right;"> 0.2421454 </td> <td style="text-align:right;"> 0 </td> <td style="text-align:right;"> 1 </td> <td style="text-align:right;"> 0 </td> </tr> <tr> <td style="text-align:right;"> 104 </td> <td style="text-align:right;"> 4 </td> <td style="text-align:right;"> -1.2128165 </td> <td style="text-align:right;"> 0 </td> <td style="text-align:right;"> 0 </td> <td style="text-align:right;"> 1 </td> </tr> <tr> <td style="text-align:right;"> 106 </td> <td style="text-align:right;"> 1 </td> <td style="text-align:right;"> -0.9736546 </td> <td style="text-align:right;"> 0 </td> <td style="text-align:right;"> 0 </td> <td style="text-align:right;"> 0 </td> </tr> <tr> <td style="text-align:right;"> 107 </td> <td style="text-align:right;"> 3 </td> <td style="text-align:right;"> 0.1843749 </td> <td style="text-align:right;"> 0 </td> <td style="text-align:right;"> 1 </td> <td style="text-align:right;"> 0 </td> </tr> <tr> <td style="text-align:right;"> 108 </td> <td style="text-align:right;"> 1 </td> <td style="text-align:right;"> -2.5231846 </td> <td style="text-align:right;"> 0 </td> <td style="text-align:right;"> 0 </td> <td style="text-align:right;"> 0 </td> </tr> <tr> <td style="text-align:right;"> 134 </td> <td style="text-align:right;"> 1 </td> <td style="text-align:right;"> 0.7839913 </td> <td style="text-align:right;"> 0 </td> <td style="text-align:right;"> 0 </td> <td style="text-align:right;"> 0 </td> </tr> <tr> <td style="text-align:right;"> 135 </td> <td style="text-align:right;"> 2 </td> <td style="text-align:right;"> 0.5787934 </td> <td style="text-align:right;"> 1 </td> <td style="text-align:right;"> 0 </td> <td style="text-align:right;"> 0 </td> </tr> <tr> <td style="text-align:right;"> 146 </td> <td style="text-align:right;"> 3 </td> <td style="text-align:right;"> 0.4955462 </td> <td style="text-align:right;"> 0 </td> <td style="text-align:right;"> 1 </td> <td style="text-align:right;"> 0 </td> </tr> <tr> <td style="text-align:right;"> 149 </td> <td style="text-align:right;"> 3 </td> <td style="text-align:right;"> 1.0877557 </td> <td style="text-align:right;"> 0 </td> <td style="text-align:right;"> 1 </td> <td style="text-align:right;"> 0 </td> </tr> <tr> <td style="text-align:right;"> 154 </td> <td style="text-align:right;"> 3 </td> <td style="text-align:right;"> NA </td> <td style="text-align:right;"> 0 </td> <td style="text-align:right;"> 1 </td> <td style="text-align:right;"> 0 </td> </tr> </tbody> </table> --- ```r mod.1 = lm(WISDOM ~ dummy_2 + dummy_3 + dummy_4, data = solomon) summary(mod.1) ``` ``` ## ## Call: ## lm(formula = WISDOM ~ dummy_2 + dummy_3 + dummy_4, data = solomon) ## ## Residuals: ## Min 1Q Median 3Q Max ## -2.6809 -0.4209 0.0473 0.6694 2.3499 ## ## Coefficients: ## Estimate Std. Error t value Pr(>|t|) ## (Intercept) -0.5593 0.1686 -3.317 0.001232 ** ## dummy_2 0.6814 0.2497 2.729 0.007390 ** ## dummy_3 0.7541 0.2348 3.211 0.001729 ** ## dummy_4 0.8938 0.2524 3.541 0.000583 *** ## --- ## Signif. codes: 0 '***' 0.001 '**' 0.01 '*' 0.05 '.' 0.1 ' ' 1 ## ## Residual standard error: 0.9389 on 111 degrees of freedom ## (5 observations deleted due to missingness) ## Multiple R-squared: 0.1262, Adjusted R-squared: 0.1026 ## F-statistic: 5.343 on 3 and 111 DF, p-value: 0.001783 ``` --- ### Interpreting coefficients When working with dummy codes, the intercept can be interpreted as the mean of the reference group. `$$\begin{aligned} \hat{Y} &= b_0 + b_1D_2 + b_2D_3 + b_3D_2 \\ \hat{Y} &= b_0 + b_1(0) + b_2(0) + b_3(0) \\ \hat{Y} &= b_0 \\ \hat{Y} &= \bar{Y}_{\text{Reference}} \end{aligned}$$` What do each of the slope coefficients mean? --- From this equation, we can get the mean of every single group. ```r newdata = data.frame(dummy_2 = c(0,1,0,0), dummy_3 = c(0,0,1,0), dummy_4 = c(0,0,0,1)) predict(mod.1, newdata = newdata, se.fit = T) ``` ``` ## $fit ## 1 2 3 4 ## -0.5593042 0.1220847 0.1948435 0.3344884 ## ## $se.fit ## 1 2 3 4 ## 0.1686358 0.1841382 0.1634457 0.1877848 ## ## $df ## [1] 111 ## ## $residual.scale ## [1] 0.9389242 ``` --- And the test of the coefficient represents the significance test of each group to the reference. This is an independent-samples *t*-test. The test of the intercept is the one-sample *t*-test comparing the intercept to 0. ```r summary(mod.1)$coef ``` ``` ## Estimate Std. Error t value Pr(>|t|) ## (Intercept) -0.5593042 0.1686358 -3.316641 0.0012319438 ## dummy_2 0.6813889 0.2496896 2.728944 0.0073896074 ## dummy_3 0.7541477 0.2348458 3.211247 0.0017291997 ## dummy_4 0.8937927 0.2523909 3.541303 0.0005832526 ``` What if you wanted to compare groups 2 and 3? --- ```r solomon = solomon %>% mutate(dummy_1 = ifelse(CONDITION == 1, 1, 0), dummy_3 = ifelse(CONDITION == 3, 1, 0), dummy_4 = ifelse(CONDITION == 4, 1, 0)) mod.2 = lm(WISDOM ~ dummy_1 + dummy_3 + dummy_4, data = solomon) summary(mod.2) ``` ``` ## ## Call: ## lm(formula = WISDOM ~ dummy_1 + dummy_3 + dummy_4, data = solomon) ## ## Residuals: ## Min 1Q Median 3Q Max ## -2.6809 -0.4209 0.0473 0.6694 2.3499 ## ## Coefficients: ## Estimate Std. Error t value Pr(>|t|) ## (Intercept) 0.12208 0.18414 0.663 0.50870 ## dummy_1 -0.68139 0.24969 -2.729 0.00739 ** ## dummy_3 0.07276 0.24621 0.296 0.76816 ## dummy_4 0.21240 0.26300 0.808 0.42104 ## --- ## Signif. codes: 0 '***' 0.001 '**' 0.01 '*' 0.05 '.' 0.1 ' ' 1 ## ## Residual standard error: 0.9389 on 111 degrees of freedom ## (5 observations deleted due to missingness) ## Multiple R-squared: 0.1262, Adjusted R-squared: 0.1026 ## F-statistic: 5.343 on 3 and 111 DF, p-value: 0.001783 ``` --- In all multiple regression models, we have to consider the correlations between the IVs, as highly correlated variables make it more difficult to detect significance of a particular X. One useful way to conceptualize the relationship between any two variables is "Does knowing someone's score on `\(X_1\)` affect my guess for their score on `\(X_2\)`?" Are dummy codes associated with a categorical predictor correlated or uncorrelated? -- ```r cor(solomon[,grepl("dummy", names(solomon))], use = "pairwise") ``` ``` ## dummy_2 dummy_3 dummy_4 dummy_1 ## dummy_2 1.0000000 -0.3306838 -0.2833761 -0.3239068 ## dummy_3 -0.3306838 1.0000000 -0.3387900 -0.3872466 ## dummy_4 -0.2833761 -0.3387900 1.0000000 -0.3318469 ## dummy_1 -0.3239068 -0.3872466 -0.3318469 1.0000000 ``` --- ### Time savers R will automatically convert factor-level variables into dummy codes -- just make sure your variable is a factor before adding it to the model! ```r class(solomon$CONDITION) ``` ``` ## [1] "integer" ``` ```r solomon$CONDITION = as.factor(solomon$CONDITION) ``` --- ```r mod.3 = lm(WISDOM ~ CONDITION, data = solomon) summary(mod.3) ``` ``` ## ## Call: ## lm(formula = WISDOM ~ CONDITION, data = solomon) ## ## Residuals: ## Min 1Q Median 3Q Max ## -2.6809 -0.4209 0.0473 0.6694 2.3499 ## ## Coefficients: ## Estimate Std. Error t value Pr(>|t|) ## (Intercept) -0.5593 0.1686 -3.317 0.001232 ** ## CONDITION2 0.6814 0.2497 2.729 0.007390 ** ## CONDITION3 0.7541 0.2348 3.211 0.001729 ** ## CONDITION4 0.8938 0.2524 3.541 0.000583 *** ## --- ## Signif. codes: 0 '***' 0.001 '**' 0.01 '*' 0.05 '.' 0.1 ' ' 1 ## ## Residual standard error: 0.9389 on 111 degrees of freedom ## (5 observations deleted due to missingness) ## Multiple R-squared: 0.1262, Adjusted R-squared: 0.1026 ## F-statistic: 5.343 on 3 and 111 DF, p-value: 0.001783 ``` --- ### Omnibus test ```r summary(mod.1) ``` ``` ## ## Call: ## lm(formula = WISDOM ~ dummy_2 + dummy_3 + dummy_4, data = solomon) ## ## Residuals: ## Min 1Q Median 3Q Max ## -2.6809 -0.4209 0.0473 0.6694 2.3499 ## ## Coefficients: ## Estimate Std. Error t value Pr(>|t|) ## (Intercept) -0.5593 0.1686 -3.317 0.001232 ** ## dummy_2 0.6814 0.2497 2.729 0.007390 ** ## dummy_3 0.7541 0.2348 3.211 0.001729 ** ## dummy_4 0.8938 0.2524 3.541 0.000583 *** ## --- ## Signif. codes: 0 '***' 0.001 '**' 0.01 '*' 0.05 '.' 0.1 ' ' 1 ## ## Residual standard error: 0.9389 on 111 degrees of freedom ## (5 observations deleted due to missingness) *## Multiple R-squared: 0.1262, Adjusted R-squared: 0.1026 *## F-statistic: 5.343 on 3 and 111 DF, p-value: 0.001783 ``` --- ### Omnibus test ```r summary(mod.2) ``` ``` ## ## Call: ## lm(formula = WISDOM ~ dummy_1 + dummy_3 + dummy_4, data = solomon) ## ## Residuals: ## Min 1Q Median 3Q Max ## -2.6809 -0.4209 0.0473 0.6694 2.3499 ## ## Coefficients: ## Estimate Std. Error t value Pr(>|t|) ## (Intercept) 0.12208 0.18414 0.663 0.50870 ## dummy_1 -0.68139 0.24969 -2.729 0.00739 ** ## dummy_3 0.07276 0.24621 0.296 0.76816 ## dummy_4 0.21240 0.26300 0.808 0.42104 ## --- ## Signif. codes: 0 '***' 0.001 '**' 0.01 '*' 0.05 '.' 0.1 ' ' 1 ## ## Residual standard error: 0.9389 on 111 degrees of freedom ## (5 observations deleted due to missingness) *## Multiple R-squared: 0.1262, Adjusted R-squared: 0.1026 *## F-statistic: 5.343 on 3 and 111 DF, p-value: 0.001783 ``` --- ### Omnibus test ```r summary(mod.3) ``` ``` ## ## Call: ## lm(formula = WISDOM ~ CONDITION, data = solomon) ## ## Residuals: ## Min 1Q Median 3Q Max ## -2.6809 -0.4209 0.0473 0.6694 2.3499 ## ## Coefficients: ## Estimate Std. Error t value Pr(>|t|) ## (Intercept) -0.5593 0.1686 -3.317 0.001232 ** ## CONDITION2 0.6814 0.2497 2.729 0.007390 ** ## CONDITION3 0.7541 0.2348 3.211 0.001729 ** ## CONDITION4 0.8938 0.2524 3.541 0.000583 *** ## --- ## Signif. codes: 0 '***' 0.001 '**' 0.01 '*' 0.05 '.' 0.1 ' ' 1 ## ## Residual standard error: 0.9389 on 111 degrees of freedom ## (5 observations deleted due to missingness) *## Multiple R-squared: 0.1262, Adjusted R-squared: 0.1026 *## F-statistic: 5.343 on 3 and 111 DF, p-value: 0.001783 ``` --- ### Omnibus test ```r anova(mod.3) ``` ``` ## Analysis of Variance Table ## ## Response: WISDOM ## Df Sum Sq Mean Sq F value Pr(>F) ## CONDITION 3 14.131 4.7105 5.3432 0.001783 ** ## Residuals 111 97.855 0.8816 ## --- ## Signif. codes: 0 '***' 0.001 '**' 0.01 '*' 0.05 '.' 0.1 ' ' 1 ``` --- class: inverse ## Next time... Analysis of Variance (the long way)